Prediction of elastic properties of short fiber reinforced composites based on machine learning
-
摘要: 短纤维增强复合材料弹性力学性能受其内部结构和基础材料性能影响显著,参数化分析这些影响需要极高的实验或数值分析成本。针对这一问题,本文将基于周期性代表性体积单元(RVE)的数值均匀化方法与人工神经网络(ANN)进行结合,分别构建了空间随机分布、层内随机分布和定向排列三种形式的短纤维增强复合材料力学性能预测代理模型。每个代理模型均可以快速实现不同参数组合(纤维长度、长径比、体积分数以及纤维和基体材料属性)下复合材料的等效弹性性能预测,拟合优度R2均在0.98以上,计算所用时间与常规模拟计算相比可忽略不计,大大节省了实验和计算成本,为短纤维增强复合材料的设计定制创造了重要条件。Abstract: The elastic and mechanical properties of short fiber reinforced composites are significantly affected by their internal structure and the properties of the underlying materials, and the parametric analysis of these effects requires extremely high experimental or numerical analysis costs. In order to solve this problem, this paper combines the numerical homogenization method based on periodic representative volume units (RVE) and artificial neural network (ANN) to construct three forms of mechanical property prediction surrogate models of short fiber reinforced composites: spatial random distribution, intralayer random distribution and aligned distribution, respectively. Each surrogate model can quickly predict the equivalent elastic properties of composites under different parameter combinations (fiber length, aspect ratio, volume fraction, and fiber and matrix material properties), and the goodness of fit R2 is above 0.98, the calculation time is negligible compared to conventional simulation calculations, which greatly saves experimental and computational costs and creates important conditions for the design and customization of short fiber-reinforced composites.
-
Key words:
- short fiber composites /
- RVE /
- parametric calculation /
- proxy model /
- elastic performance prediction
-
图 11 Csf/Mg复合材料弹性力学性能预测的拟合回归图:(a) 纤维在空间随机分布;(b) 纤维在层内随机分布;(c) 纤维定向分布
Figure 11. Fitting regression diagram for prediction of elastic mechanical properties of Csf/Mg composites: (a) The fibers are randomly distributed in space; (b) The fibers are randomly distributed in layer; (c) The fibers are aligned in Z direction
表 1 数值均匀化、Digimat和拉伸试验[20]得到的Csf/Mg复合材料等效弹性性能比较
Table 1. Comparison of effective elastic properties of Csf/Mg composite obtained from the numerical homogenization, Digimat and tensile experiment[20]
Properties Numerical homogenization Digimat Tensile experiment ErrorN-T ErrorD-T E/GPa 49.42 52.98 50.45 2.0% 5.0% G/GPa 18.12 19.44 19.02 4.7% 2.2% ν 0.3430 0.3384 0.3425 0.1% 1.2% 表 2 计算结果与Liu[10]的工作和Digimat的比较
Table 2. Comparison of the computational results with Liu’s[10] work and Digimat
Properties Random distribution in layer Aligned distribution Present work Liu’s work Digimat Present work Liu’s work Digimat E11/GPa 2.8648 2.8176 2.7382 3.0210 3.4347 2.9302 E22/GPa 2.6166 2.7453 2.5915 3.1762 3.4951 2.9302 E33/GPa 2.1908 2.1695 2.0903 17.933 19.712 20.540 G12/GPa 1.0134 1.0467 0.99472 1.0272 1.1591 0.98722 G13/GPa 0.74077 0.74028 0.71036 1.1158 1.3543 1.0744 G23/GPa 0.73648 0.73819 0.70854 1.2524 1.3049 1.0744 ν12 0.34793 0.33629 0.34558 0.4407 0.42266 0.48406 ν21 0.31873 0.32766 0.32706 0.4634 0.43010 0.48406 ν13 0.33177 0.34107 0.34547 0.053278 0.055075 0.045703 ν31 0.25533 0.26263 0.26372 0.31627 0.31608 0.32037 ν23 0.34807 0.34568 0.35505 0.054968 0.054838 0.045703 ν32 0.29293 0.27318 0.28638 0.31035 0.30928 0.32037 表 3 选定变量及其取值范围
Table 3. Input variables and their value ranges
lf/mm af Vf/% Ef/GPa νf Em/GPa νm Random distribution in space 1-5 5-15 5-10 70-230 0.20-0.35 0.1-6 0.30-0.45 Random distribution in layer 1-5 10-15 5-10 70-230 0.20-0.35 0.1-6 0.30-0.45 Aligned distribution 1-10 20-40 20-30 70-230 0.20-0.35 0.1-6 0.30-0.45 Notes: lf—Length of fiber; af—Aspect ratio of fiber; Vf—Volume fraction of fiber; Ef—Young’s modulus of fiber; νf—Poisson’s ratio of fiber; Em—Young’s modulus of matrix; νm—Poisson’s ratio of matrix. -
[1] WAN Y, TAKAHASHI J. Tensile and compressive properties of chopped carbon fiber tapes reinforced thermoplastics with different fiber lengths and molding pressures[J]. Composites Part A:Applied Science and Manufacturing, 2016, 87: 271-281. doi: 10.1016/j.compositesa.2016.05.005 [2] KAMMOUN S, DOGHRI I, ADAM L, et al. First pseudo-grain failure model for inelastic composites with misaligned short fibers[J]. Composites Part A:Applied Science and Manufacturing, 2011, 42(12): 1892-1902. doi: 10.1016/j.compositesa.2011.08.013 [3] KAMMOUN S, DOGHRI I, BRASSART L, et al. Micromechanical modeling of the progressive failure in short glass–fiber reinforced thermoplastics–First Pseudo-Grain Damage model[J]. Composites part A:applied science and manufacturing, 2015, 73: 166-175. doi: 10.1016/j.compositesa.2015.02.017 [4] REZAEI F, YUNUS R, IBRAHIM N A. Effect of fiber length on thermomechanical properties of short carbon fiber reinforced polypropylene composites[J]. Materials & Design, 2009, 30(2): 260-263. [5] CHOU T W, NOMURA S, TAYA M. A self-consistent approach to the elastic stiffness of short-fiber composites[J]. Journal of Composite Materials, 1980, 14(3): 178-188. doi: 10.1177/002199838001400301 [6] MORI T, TANAKA K. Average stress in matrix and average elastic energy of materials with misfitting inclusions[J]. Acta metallurgica, 1973, 21(5): 571-574. doi: 10.1016/0001-6160(73)90064-3 [7] HASHIN Z, SHTRIKMAN S. On some variational principles in anisotropic and nonhomogeneous elasticity[J]. Journal of the Mechanics and Physics of Solids, 1962, 10(4): 335-342. doi: 10.1016/0022-5096(62)90004-2 [8] HASHIN Z, SHTRIKMAN S. A variational approach to the theory of the elastic behaviour of multiphase materials[J]. Journal of the Mechanics and Physics of Solids, 1963, 11(2): 127-140. doi: 10.1016/0022-5096(63)90060-7 [9] AFFDL J C H, KARDOS J L. The Halpin-Tsai equations: a review[J]. Polymer Engineering & Science, 1976, 16(5): 344-352. [10] LIU H, ZENG D, LI Y, et al. Development of RVE-embedded solid elements model for predicting effective elastic constants of discontinuous fiber reinforced composites[J]. Mechanics of materials, 2016, 93: 109-123. doi: 10.1016/j.mechmat.2015.10.011 [11] IONITA A, WEITSMAN Y J. On the mechanical response of randomly reinforced chopped-fibers composites: Data and model[J]. Composites science and technology, 2006, 66(14): 2566-2579. doi: 10.1016/j.compscitech.2006.01.019 [12] NAZARENKO L, STOLARSKI H, ALTENBACH H. Effective properties of short-fiber composites with Gurtin-Murdoch model of interphase[J]. International Journal of Solids and Structures, 2016, 97: 75-88. [13] KARI S, BERGER H, RODRIGUEZ-RAMOS R, et al. Computational evaluation of effective material properties of composites reinforced by randomly distributed spherical particles[J]. Composite structures, 2007, 77(2): 223-231. doi: 10.1016/j.compstruct.2005.07.003 [14] WURKNER M, BERGER H, GABBERT U. Numerical investigations of effective properties of fiber reinforced composites with parallelogram arrangements and imperfect interface[J]. Composite Structures, 2014, 116: 388-394. doi: 10.1016/j.compstruct.2014.05.012 [15] HARPER L T, QIAN C, TURNER T A, et al. Representative volume elements for discontinuous carbon fibre composites–Part 1: Boundary conditions[J]. Composites Science and Technology, 2012, 72(2): 225-234. doi: 10.1016/j.compscitech.2011.11.006 [16] HARPER L T, QIAN C, TURNER T A, et al. Representative volume elements for discontinuous carbon fibre composites–Part 2: Determining the critical size[J]. Composites Science and Technology, 2012, 72(2): 204-210. doi: 10.1016/j.compscitech.2011.11.003 [17] JAIN A, LOMOV S V, ABDIN Y, et al. Pseudo-grain discretization and full Mori Tanaka formulation for random heterogeneous media: Predictive abilities for stresses in individual inclusions and the matrix[J]. Composites science and technology, 2013, 87: 86-93. doi: 10.1016/j.compscitech.2013.08.009 [18] FLIEGENER S. Micromechanical finite element modeling of long fiber reinforced thermoplastics[D]. Karlsruhe, Karlsruher Institut für Technologie (KIT), Diss. , 2015, 2015. [19] LU Z, YUAN Z, LIU Q. 3D numerical simulation for the elastic properties of random fiber composites with a wide range of fiber aspect ratios[J]. Computational materials science, 2014, 90: 123-129. doi: 10.1016/j.commatsci.2014.04.007 [20] QI L, TIAN W, ZHOU J. Numerical evaluation of effective elastic properties of composites reinforced by spatially randomly distributed short fibers with certain aspect ratio[J]. Composite Structures, 2015, 131: 843-851. doi: 10.1016/j.compstruct.2015.06.045 [21] TIAN W, QI L, ZHOU J, et al. Representative volume element for composites reinforced by spatially randomly distributed discontinuous fibers and its applications[J]. Composite Structures, 2015, 131: 366-373. doi: 10.1016/j.compstruct.2015.05.014 [22] MAHDAVI M, YOUSEFI E, BANIASSADI M, et al. Effective thermal and mechanical properties of short carbon fiber/natural rubber composites as a function of mechanical loading[J]. Applied Thermal Engineering, 2017, 117: 8-16. doi: 10.1016/j.applthermaleng.2017.02.004 [23] DUSCHLBAUER D, BÖHM H J, PETTERMANN H E. Computational simulation of composites reinforced by planar random fibers: homogenization and localization by unit cell and mean field approaches[J]. Journal of Composite Materials, 2006, 40(24): 2217-2234. doi: 10.1177/0021998306062317 [24] KARI S, BERGER H, GABBERT U. Numerical evaluation of effective material properties of randomly distributed short cylindrical fibre composites[J]. Computational materials science, 2007, 39(1): 198-204. doi: 10.1016/j.commatsci.2006.02.024 [25] OGIERMAN W, KOKOT G. A study on fiber orientation influence on the mechanical response of a short fiber composite structure[J]. Acta Mechanica, 2016, 227(1): 173-183. doi: 10.1007/s00707-015-1417-0 [26] BABU K P, MOHITE P M, UPADHYAY C S. Development of an RVE and its stiffness predictions based on mathematical homogenization theory for short fibre composites[J]. International Journal of Solids and Structures, 2018, 130: 80-104. [27] LECLERC W, KARAMIAN-SUEVILLE P, Vivet A. An efficient and automated 3D FE approach to evaluate effective elastic properties of overlapping random fibre composites[J]. Computational Materials Science, 2015, 99: 1-15. doi: 10.1016/j.commatsci.2014.10.047 [28] KOHONEN T. The 'neural' phonetic typewriter[J]. Computer, 1988, 21(3): 11-22. doi: 10.1109/2.28 [29] ABADI M, AGARWAL A, BARHAM P, et al. Tensorflow: Large-scale machine learning on heterogeneous distributed systems[J]. arXiv preprint arXiv:1603, 0446, 7: 2016. [30] KETKAR N, MOOLAYIL J, KETKAR N, et al. Introduction to pytorch[J]. Deep Learning with Python: Learn Best Practices of Deep Learning Models with PyTorch, 2021: 27-91. [31] LIU X, TIAN S, TAO F, et al. A review of artificial neural networks in the constitutive modeling of composite materials[J]. Composites Part B:Engineering, 2021, 224: 109152. doi: 10.1016/j.compositesb.2021.109152 [32] HILL R. Elastic properties of reinforced solids: some theoretical principles[J]. Journal of the Mechanics and Physics of Solids, 1963, 11(5): 357-372. doi: 10.1016/0022-5096(63)90036-X [33] LOH W L. On Latin hypercube sampling[J]. The Annals of Statistics, 1996, 24(5): 2058-2080. [34] DRUGAN W J, WILLIS J R. A micromechanics-based nonlocal constitutive equation and estimates of representative volume element size for elastic composites[J]. Journal of the Mechanics and Physics of Solids, 1996, 44(4): 497-524. doi: 10.1016/0022-5096(96)00007-5 [35] KANIT T, FOREST S, GALLIET I, et al. Determination of the size of the representative volume element for random composites: statistical and numerical approach[J]. International Journal of solids and structures, 2003, 40(13-14): 3647-3679. doi: 10.1016/S0020-7683(03)00143-4 [36] IORGA L, PAN Y, PELEGRI A. Numerical characterization of material elastic properties for random fiber composites[J]. Journal of Mechanics of materials and Structures, 2008, 3(7): 1279-1298. doi: 10.2140/jomms.2008.3.1279 [37] PELISSOU C, BACCOU J, MONERIE Y, et al. Determination of the size of the representative volume element for random quasi-brittle composites[J]. International Journal of Solids and Structures, 2009, 46(14-15): 2842-2855. doi: 10.1016/j.ijsolstr.2009.03.015 [38] GITMAN I M, ASKES H, SLUYS L J. Representative volume: Existence and size determination[J]. Engineering fracture mechanics, 2007, 74(16): 2518-2534. doi: 10.1016/j.engfracmech.2006.12.021 [39] KANIT T, N’GUYEN F, FOREST S, et al. Apparent and effective physical properties of heterogeneous materials: Representativity of samples of two materials from food industry[J]. Computer Methods in Applied Mechanics and Engineering, 2006, 195(33-36): 3960-3982. doi: 10.1016/j.cma.2005.07.022 [40] PENG G C Y, ALBER M, BUGANZA T A, et al. Multiscale modeling meets machine learning: What can we learn?[J]. Archives of Computational Methods in Engineering, 2021, 28: 1017-1037. doi: 10.1007/s11831-020-09405-5 [41] JABBAR H, KHAN R Z. Methods to avoid over-fitting and under-fitting in supervised machine learning (comparative study)[J]. Computer Science, Communication and Instrumentation Devices, 2015, 70(10.3850): 978-981. -
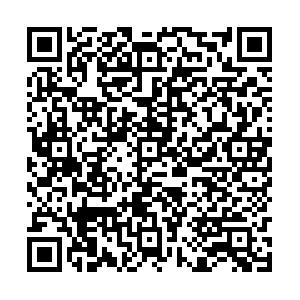
计量
- 文章访问数: 151
- HTML全文浏览量: 82
- 被引次数: 0