Research progress on mixture optimization of concrete based on machine learning and metaheuristic algorithms
-
摘要: 混凝土配合比决定其成本、工作性能、力学性能和耐久性能,传统混凝土配合比优化方法是通过大量实验室试验,需要消耗大量时间、人力和资源。为了解决上述问题,利用机器学习和元启发式优化算法进行混凝土配合比优化已被证明是一种具有广阔前景的技术手段。本文全面回顾了有关混凝土配合比设计和优化方面的研究。首先,讨论了常用的机器学习和元启发式算法的基本工作原理和优势。然后,归纳总结了基于机器学习和元启发式算法在单一目标、多目标优化各种类型混凝土配合比方面的应用。最后,结合当前的技术水平,强调并讨论了推进混凝土配合比设计和优化领域的当前趋势和机遇,为机器学习技术在混凝土领域的更深层次的开发和应用提供了依据。Abstract: The concrete mix ratio determines its cost, workability, mechanical properties, and durability. The traditional method of concrete mix ratio optimization is through a large number of laboratory tests, which consumes a lot of time, labor, and resources. To solve the above problems, concrete proportion optimization using machine learning and meta-heuristic optimization algorithms has been proven to be a promising technical tool. Presents a comprehensive review of the research on concrete proportion design and optimization. First, the basic working principles and advantages of commonly used machine learning and meta-heuristic algorithms are discussed. Then, the applications of machine learning and meta-heuristic-based algorithms in single-objective and multi-objective optimization of various types of concrete proportions are summarized. Finally, current trends and opportunities in advancing the field of concrete proportion design and optimization are highlighted and discussed in the context of the current state of the art, providing a basis for deeper development and application of machine learning techniques in the field of concrete.
-
Key words:
- Concrete mixture /
- Machine learning /
- Metaheuristic algorithm /
- Optimization
-
表 1 四种元启发式优化算法对比分析
Table 1. Comparative analysis of four meta-heuristic optimization algorithms
Algorithm Author Year Inspiration Class Application area Genetic Algorithm Holland and Reitman 1978 The process of natural selection Evolutionary-based Power system, image reconstruction, scheduling, engineering, routing, feature selection, filtering recommender system, travelling salesman problem, electromagnetic optimization. Particle swarm optimization Eberhart and Kennedy 1995 The process of bird flock Swarm-based Feature selection, job scheduling, financial time series forecast, vapor-liquid equilibrium problem, engineering, economic dispatch problem, scheduling problem, medical diagnosis. Beetle Antennae Search Xiangyuan Jiang and Shuai Li 2017 The process of the searching behavior of longhorn beetles. Individual-based Power system, image reconstruction, feature selection, job scheduling, engineering, scheduling problem, electromagnetic optimization. Artificial Bee Colony Karaboga and Basturk 2007 The process of honey harvesting behavior of bee colony Swarm-based Scheduling, estimation of transportation energy demend, forecasting the blast-produced ground virbration, travelling salesman problem, engineering problem, clustering. 表 2 应用机器学习(ML)优化普通混凝土配合比
Table 2. Optimal concrete mixture based on machine learning (ML)
Algorithms Input variables Output variables Optimization objectives Evaluation metrics Datasets Year Authors BPNN Cement, fine/coarse aggregate, water, superplasticizer Compressive strength, slump, permeability coefficient, elastic modulus / / 117 2003 Liu et al[21] ANN Weathered sand, soil, cement, water, superplasticizer Compressive strength, tangent modulus / / 10 2004 Li et al[22] ANN Cement, fly ash, fine and coarse aggregates, water, superplasticizer, reducing-water ratio of superplasticizer Strength, slump / Average sum-squared error 18 2006 Ji et al[23] BPNN, GA Cement, fine/coarse aggregate, fly ash, superplasticizer Compressive strength, slump / Average relative error 150 2007 Chen et al[24] NN, GA Cement, fly ash, slag, water, superplasticizer, fine and coarse aggregates, binder, water/cement, water/binder water/solid, superplasticizer /binder, fly ash/binder, slag/binder, pozzolans/binder aggregate/binder, fine aggregate /aggregate Strength, slump / RMSE 103 2007 Yeh[25] GA, ANN, BPNN Cement, fly ash, fine aggregate, coarse aggregate, admixture, water-binder ratio. Slump / RMSE, MAPE, R 560 2015 Chandwani et al[26] BBP, Regression Cement, water, silica fume, coarse aggregate, fine aggregate, superplasticizer, the maximum size of aggregate and concrete age Compressive strength / RMSE 1030 2019 Golafshani et al[27] DNN Cement, water, fine-grained aggregate, coarse aggregate, water-cement ratio Compressive strength / MAE, MSE, RMSE 741 2021 Ziolkowski et al[28] ExGBT, LGBT, GAM, KDP, Anomaly detection algorithm, K-means algorithm Water, cement, fly ash, water-reducing admixture, air-entraining admixture, coarse/fine aggregate, fresh air Fire-induced spalling, chloride penetration, strength / Area under the ROC curve, Log loss error 10000 2022 Naser[30] DL, GP, ANN, BO Mixture proportions, chemical composition, particle size distribution, type of specimen, curing time Compressive strength / RMSE, MAE, MAD, R2 760 2023 Mahjoubi et al[31] LR, NN, BT, RF, SVM Water/cement, cement, fly ash contents, water-reducing admixture, air-entraining admixture contents, coarse/fine aggregate contents, fresh air content Compressive strength Compressive strength, cost, embodied CO2 RMSE, R2, MAPE 1031/
99942019 Young et al[32] WCA, SLC, GA, ANN, SVM, Regression Age, cement, water, superplasticizer, coarse aggregate, fine aggregate, the ratios of water to binder, superplasticizer to binder, fine aggregate to total aggregate, coarse aggregate to binder Compressive strength Compressive strength, cost, CO2 emission, energy, resource consumptions MAE, R, R2, MSE, RMSE 232 2020 Naseri et al[34] BPNN, BAS, SVM, LR, DT, MLR, KNN, RF, NSGA-II, MOEA, MOPSO Ordinary Portland cement, silica fume, water, coarse/fine aggregate, maximum size of coarse aggregate, superplasticizer, curing age Strength Strength, cost, embodied CO2 RMSE, R 1030 2021 Zhang et al[11] RF, DT, SVM, BAS Cement, water, blast furnace slag, coarse/fine aggregate, superplasticizer Uniaxial compressive strength Uniaxial compressive strength, cost RMSE, R 102 2022 Zhu et al[35] COP, WCP, DL Age, water, cement, fine/coarse aggregate, superplasticizer, supplementary cementing materials, weight ratios of water to binder, supplementary cementing materials to binder, coarse aggregate to binder, fine aggregate to total aggregate, superplasticizer to binder Compressive strength Compressive strength, cost, emission-CO2, energy consumption, material consumption R, MSE, MAE, R2, RMSE 1200 2023 Naseri et al[36] Elastic net, Extremely randomized tree, Lasso/Ridge /amma regression, RF, DT, AdaBoost, GB, ExGBT, LightGBM, CatBoost, NSGA-III, C-TAEA Water, cement, mineral admixture, fine/coarse aggregate, chemical admixture, performance parameter Compressive strength,
binder intensityCompressive strength,
binder intensity, costR2, RMSE, MAE, MGD 610 2023 Zheng et al[37] Notes: DNN - Deep neural network, ExGBT - Extreme gradient boosted tree, LGBT - Light gradient boosted tree, GAM - Generalized additive model, KDP - Keras deep residual neural network, DL - Deep learning, GP - Gaussian processes, BO - Bayesian optimization, LR – Linear regression, BT – Boosted tree, WCA - Water cycle algorithm, SLC - Soccer league competition, RMSE - Root mean square error, MAPE - Mean absolute percentage error, MAE - Mean absolute error, MSE - Mean square error, ROC - Receiver operating characteristic, MAD - Median absolute deviation, DT - Decision tree, MLR - Multivariable linear regression, KNN - K-nearest neighbours, MOEA-, MOPSO - Multi-objective particle swarm optimization, COP - Coyote optimization programming, WCP - Water cycle programming, GB - Gradient boosting, C-TAEA - Constrained-targeted adaptive evolutionary algorithm, MGD - Mean gamma deviance. 表 3 应用ML优化UHPC/HPC/HSC配合比
Table 3. Optimal UHPC/HPC/HSC mixture based on machine learning
Algorithms Input variables Output variables Optimization
objectivesEvaluation metrics Datasets Year Authors ANN Cement, fly ash, blast furnace slag, water, superplasticizer, coarse/fine aggregate, water/cement ratio, water/cementitious ratio, superplasticizer/cementitious ratio, fly ash/cementitious ratio, slag/cementitious ratio (fly ash+ slag)/cementitious ratio Compressive strength, slump / R2 695/
1201999 Yeh[33] GA Water to binder ratio, water, fine aggregate ratio, fly ash replacement ratio, silica fume replacement ratio, air-entraining agent, superplasticizer Compressive strength, slump / R2 189 2004 Lim et al[38] Elitist GA Cement, fly ash, fine/coarse aggregates, water, superplasticizer, water / cement, water / (cement + fly ash), fly ash / cement, fly ash / (cement +fly ash) Compressive strength / MSE 350 2009 Jayaram et al[39] Harmony search algorithm, NN, GA Cement, fly ash, silica fume, fine/coarse aggregates, water, superplasticizer, fine aggregate ratio, water / binder Compressive strength, slump / Probability 189 2012 Lee et al[40] GA, ESVM Cement; fly ash; blast furnace slag; water; superplasticizer; coarse/fine aggregate, age of testing Compressive strength / RMSE, MAE 1030 2014 Cheng[41] GP Cement, blast furnace slag, fly ash, water, superplasticizer, coarse/fine aggregate Strength / RMSE, Pearson correlation coefficient 453 2021 Ke et al[42] SOS, LSSVM, BPNN, ESIM, SVM Fine/coarse aggregate, fly ash, silica fume, cement, blast furnace slag, water, superplasticizer, age of testing Compressive strength, slump, electrical
resistivityCompressive strength, slump, electrical
resistivity, costR, RMSE, MAPE, MAE 597/
282/
2642023 Cheng et al[43] BPNN, GA Cement,water/cement,silica fume,superplasticizer,fine/coarse aggregate,steel fiber content Compressive strength / / 50 2020 Chen et al[44] GA, ANN Cement, silica fume, limestone powder, river sand; water/cementitious materials ratio, superplasticizer/cementitious materials ratio Packing density Workability, packing density, compressive strength, pore structure, economy, ecology R2, RDP, RMSE, MAPE 26 2020 Fan et al[45] GA, ANN, LR Cement, limestone powder, silica fume, river sand Compressive strength, packing density / R2, RDP, RMSE 80 2021 Fan et al[46] AdaBoost, LightGBM, XGBoost, CatBoost, RF Cement, silica fume, quartz sand, steel fiber, superplasticizer, water, concrete age Compressive strength / RMSE, R2, MAE 400 2023 Zou et al[47] RF, CART Cement, silica fume, supplementary cementing materials, quartz powder, water, superplasticizer proportion, siliceous micro sand, maximum size of the sand’s particle, water to binder, mixture’s total fiber volume, total fiber reinforcement index Energy absorption capacity, strain at peak stress / R2 600 2023 Garcia et al[48] ANN Fly ash, silica fume, superplasticizer, water/cement, water to binder Compressive strength / / 9 2004 Wang et al[49] ANN, GA Water to binder ratio, sand ratio, the amount of cement per cubic meter of concrete, the ratio of fly ash/slag/silica fume mass to cementitious materials mass Slump, compressive strength, tensile strength, elastic modulus, shrinkage, creep coefficient, cracking risk coefficient Slump, compressive strength, tensile strength, elastic modulus, shrinkage, creep coefficient, cracking risk coefficient MAE, MSE, RMSE, R2 25 2020 Li et al[50] BPNN, SVR, RT, RF, KNN, LR, MLR, PSO Cement, blast furnace slag, fly ash, water, fine/coarse aggregate, bentonite, silty clay, superplasticizer, curing age Strength, slump Strength, slump, cost RMSE, R 1030 2020 Zhang et al[51] RF, NSGA-II Water to binder, cement, fine/coarse aggregate, fly ash, silica fume, superplasticizer Compressive strength, chloride ion permeability coefficient Compressive strength, chloride ion permeability coefficient, cost RMSE, R2 71 2022 Wu et al[52] RF, AEPSO Cement, blast furnace slag, fly ash, water, fine/coarse aggregate, superplasticizer, age Compressive strength Compressive strength, cost, emission-CO2 RMSE, R, MAE 1133 2022 Ren et al[53] RF, NSGA-II, BPNN, SVM, GBDT Cement, fine/coarse aggregate, superplasticizer, fly ash, water-binder ratio Relative dynamic elastic modulus, chloride ion permeability coefficient, cost Relative dynamic elastic modulus, chloride ion permeability coefficient, cost RMSE, R2, MAPE 92 2023 Chen et al[54] RF, RFE, BO, LSSVM, NSGA-III, BPNN, WNN Fly ash, cement, fine/coarse aggregate, water, composite superplasticizer, water-binder ratio, average particle size, sand ratio, mud, needle-like particles, water absorption Chloride ion permeability coefficient, relative dynamic elastic modulus, strength Chloride ion permeability coefficient, relative dynamic elastic modulus, strength, cost RMSE, R2 100 2023 Yang et al[55] MLR, MLPNN, GPR, PSO Cement, silica fume, quartz flour, siliceous sand, water, polycarboxylate ether-based superplasticizers, steel microfibers Strength, slump Strength, slump, cost RMSE, R, MAE 53 2022 Sadrossadat et al[56] GP, BBO, LR, RF, XGBoost, GA-ANN Cement, water, sand, admixture, quartz powder, steel fiber, silica fume, fly ash Compressive strength Compressive strength, workability, embodied-CO2, packing density, cost MSE, R2 110 2022 Saleh et al[57] Ridge, MLP, SVM, Partial least squares, RF, BO, LightGBM, AGE-MOEA, XGBoost, Extra trees, C-TAEA, NSGA-II, UNSGA-III Portland cement, fly ash, slag, silica fume, metakaolin, nano silica, limestone powder, quartz powder, fine sand, tap water, superplasticizer, steel fiber Compressive strength, flexural strength, mini-slump spread, porosity Compressive strength, flexural strength, mini-slump spread, porosity, life-cycle carbon footprint, embodied energy, material cost MAE, MAPE, MAD, R2 785 2023 Mahjoubi et al[58] XGBoost, SVR, GBR, RFR, GA, NSGA-II Cement, fly ash, blast furnace slag, superplasticizer, water, coarse/fine aggregate, age Compressive strength Compressive strength, cost, CO2 emission R2, RMSE, MAE 1342+1133 2023 Tipu et al[59] Notes: ESVM - Evolutionary support vector machine, SOS - Symbiotic organism search, LSSVM - Least squares support vector machine, ESIM - Evolutionary support vector machine inference model, CART - Classification and regression tree, RT – Regression tree, AEPSO - Adaptive evolutionary particle swarm optimization, GBDT - Gradient boosting decision tree, RFE - Recursive feature elimination, WNN - Wavelet neural network, MLPNN - Multi-layer perceptron neural network, GPR - Gaussian processing regression, BBO - Batch bayesian optimization, MLP - Multilayer perceptron, AGE-MOEA - Adaptive geometry estimation-based many-objective evolutionary algorithm, GBR - Gradient boosting regression, RFR – Random forest regression, RDP - Relative percent deviation. 表 4 应用ML优化RAC/RBAC配合比
Table 4. Optimal RAC/RBAC mixture based on machine learning
Algorithms Input variables Output variables Optimization
objectivesEvaluation metrics Datasets Year Authors GA Water/binder, water, cement, sand, aggregate, admixture Slump, compressive strength, final setting, specific gravity, elastic modulus, carbonation speed coefficient, price, CO2 emission Slump, compressive strength, final setting, specific gravity, elastic modulus, carbonation speed coefficient, price, CO2 emission Probability 6/4 2013 Park[60] NN Cement, water/cement, crushed tile, crushed brick, natural aggregate Compressive strength / MAE, RMSE, MAPE, R, E 147 2017 Šipoš[61] ICA, MLP, GA, PSO Water, cement, recycled coarse aggregates, natural coarse/fine aggregates, water/cement Compressive, tensile, flexural strengths, slump / MAE 1348 2021 Pajand et al[62] GBRT, PSO, RNN, GP Water/cement, cement, sand, recycled aggregate, gavel, superplasticizer, silica fume, age, specimen type Compressive strength Compressive strength, cost, CO2 emission RMSE, MAE, R2 1134 2020 Nunez et al[63] FA, RF, BPNN, SVM Cement, water, sand, natural coarse aggregates, superplasticizer, recycled coarse aggregates, maximum size, water absorption, density Uniaxial compressive strength, splitting tensile strength Uniaxial compressive strength, splitting tensile strength, cost, CO2 emission RMSE, R 344 2020 Zhang et al[64] BPNN, GWO, SVM, RF, ELM, XGBoost, GRNN, PSO Cement, water/cement, crushed tile ratio, crushed brick ratio, natural aggregate ratio Compressive strength Compressive strength, cost, CO2 emission R, R2, SD, RMSE 182 2023 Wang et al[65] BPNN, GPR, RF, BO, GBDT, NSGA-II Cement,water, sand, fly ash, aggregate replacement ratio, aggregate water absorption, age, carbon dioxide volume fraction, ambient temperature, humidity, carbonation time Electrical flux, carbonization depth Electrical flux, carbonation depth, cost, strength R2, RMSE 200+500 2023 Liu et al[66] BPNN, GPR, CART, RF, BO, GBDT, XGBoost, CMOPSO Water, cement, sand, coarse aggregates, fly ash, strength grade of cement, replacement ratio of recycled coarse aggregates, weighted water absorption of coarse aggregates, maximum particle size of coarse aggregates, curing time Compressive strength Compressive strength, materials cost, carbon footprint, energy intensity R2, RMSE, MAPE 1373 2023 Liu et al[67] Notes: ICA – Imperialist competitive algorithm, GBRT – Gradient boosting regression tree, RNN – Recurrent neural network, FA – Firefly algorithm, GWO – Gray wolf optimizer, ELM – Extreme learning machine, GRNN – Generalized regression neural network, CMOPSO - Competitive mechanism-based multi-objective particle swarm optimization, SD - Standard deviation. 表 5 应用ML优化GPC/AAC配合比
Table 5. Optimal GPC/AAC mixture based on machine learning
Algorithms Input variables Output variables Optimization
objectivesEvaluation metrics Datasets Year Authors MARS Fly ash, aggregates, alkaline activator, added water, NaOH molarity, solid% in Na2SiO3, heat curing Compressive strength / R, RMSE, MAE 98 2018 Lokuge et al[68] ANN Fly ash, coarse aggregate, fine aggregate, NaOH activator, Na2SiO3 activator, added water, SiO2 % in Na2SiO3, Na2O % in Na2SiO3, NaOH molarity, heat curing Compressive strength, flexural strength, elastic modulus / MSE, R, 166 2021 Gunasekara et al[69] RF Precursor, ground granulated blast furnace slag ratio, Na2O, elastic modulus, water, fine/coarse aggregate Compressive strength, slump, static/dynamic yield stress, plastic viscosity Compressive strength, slump, static/dynamic yield stress, plastic viscosity, CO2 emission R2, MAE, MAPE, RMSE 145+193 2023 Sun et al[70] ANN, GA Total mass of SiO2, Al2O3, Fe2O3, CaO, Na2O, fine/coarse aggregates, superplasticizer, water, age, curing temperature, time, relative humidity Compressive strength, slump Transportation, CO2 emission, cost RMSE, MAE, R2, R 1178 2022 Shobeiri et al[71] NSGA-II, BPNN, XGBoost Fly ash chemical composition, superplasticizer; fly ash; fine/coarse aggregate; extra water; high temperature; high temperature curing; standard curing Uniaxial compressive strength Uniaxial compressive strength, cost, CO2 emission MSE, MAE, R2 896 2023 Dong et al[72] RFR, ETR, GBR, XGBR, BO, NSGA-II Fly ash, blast furnace slag, sodium silicate, sodium hydroxide, water, coarse/fine aggregate, curing relative humidity, temperature, curing age, silica modulus, reactivity modulus, alumina modulus, lime modulus, hydraulic modulus Uniaxial compressive strength Uniaxial compressive strength, cost, CO2 emission R, MAE, RMSE 676 2023 Huang et al[73] RF, GB, BPNN, GPR, PSO Slag, fly ash, coarse/fine aggregate, NaOH, sodium silicate, water, superplasticizer Compressive strength Compressive strength, cost, carbon emission R2, RMSE, MAPE 143 2023 Li et al[74] Notes: RFR – Random forest regression, ETR – Extremely randomized tree, XGBR – Extreme gradient boosting regression. 表 6 应用ML优化LWC配合比
Table 6. Optimal LWC mixture based on machine learning
Algorithms Input variables Output variables Optimization objectives Evaluation metrics Datasets Year Authors SVR, MLP, GBR, XGBoost Cement, supplementary cementitious materials, light weight aggregates types, normal weight aggregates, superplasticizer, age of testing, oven dry densities of each light weight high strength concrete mix Compressive strength, splitting tensile strength / R2, RMSE, MAE, MAPE 403 2023 Sifan et al[75] SVM, ANN, DT, GPR, XGBoost Cement, sand, water-to-cement ratio, lightweight aggregate, normal aggregate, density of lightweight aggregate, water absorption of lightweight aggregate, superplasticizer, curing time, fly ash, lightweight aggregate type Compressive strength / RMSE, MAE, MSE, R2, 420 2023 Hussain et al[76] ANN, DNN, RBM, DBN, GA, NSGA-II The percentage of lightweight expanded clay aggregate, water-to cement ratio, cement content, silica fume dosage Compressive, tensile strength Compressive strength, cost, Life cycle assessment R2, MAE, RMSE 240 2021 Dabbaghi et al[77] MOFA, SVR Cement, water, natural fine aggregate, lightweight coarse aggregate, superplasticizer, the density of lightweight coarse aggregate, maximum size of coarse aggregate, age Uniaxial compressive strength, density Uniaxial compressive strength, density, cost RMSE, MAE, MAPE, R 144 2021 Zhang et al[78] Notes: RBM – Restricted Boltzmann machine, MOFA – Multi-objective firefly algorithm. 表 7 应用ML优化其它类型混凝土配合比
Table 7. Optimal other types concrete mixture based on machine learning
Concrete types Algorithms Input variables Output variables Optimization
objectivesEvaluation metrics Datasets Year Authors Pozzolanic concrete ANN, BPNN Water, cement, ground granulated blast furnace slag, fly ash, coarse/fine aggregate, superplasticizer Compressive strength, slump / R2, SSE 482/
2952018 Kao et al[79] Cemented paste backfill PSO, MR Cement percentage, solid content, water contents Strength, yield stress, cost Strength, yield stress, cost R2 16 2020 Sadrossadat et al[80] Wet-sprayed concrete ANN, PSO, GA Cement, water-cement ratio, water-reducing agent, sand/gravel ratio, age of maintenance Compressive strength / R2, MRE 32 2021 Han et al[81] Cement grouting material AdaBoost Water-cement ratio, expansion agent, water reducing agent, accelerating agent Flexural, compressive strengths, fluidity, shrinkage rate / R2 16 2022 Ren et al[82] Steelmaking slag concrete SVR, ANN, XGBoost, GPR Cement, fine steel slag, coarse steel slag, fine/coarse aggregate; water, superplasticizer, pozzolanic admixtures, supplementary cementing materials, filler Compressive strength / R2, MAE, RMSE 406/
1462022 Penido et al[83] Cement-based grouting materials SVR, DT, RF, AdaBoost, XGBoost, BO Water-binder ratio, sand-binder ratio, silica fume, blast furnace slag, metakaolin, fly ash, hardening expansion agent, superplasticizer, plastic expansion agent, stabilizer, anti-foaming agent, early strength agent Compressive strength, fluidity / R2, RMSE 442/
2172023 Zhao et al[84] Aerogel-incorporated concrete DT, SVR, MLP, RF, XGBoost, GBDT, LightGBM Water-binder ratio, aerogel replacement rate, silica fume replacement rate, age, dry/saturated state Compressive/
flexural strengths, thermal conductivity/ R2, MAE, RMSE, MAPE 660 2023 Han et al[85] Pervious concrete SVR, BAS Water-to-cement, coarse aggregate size, aggregate-to-cement ratio Permeability coefficient, uniaxial compressive strength, splitting tensile strength / R, RMSE 90 2020 Zhang et al[86] Steel fibre reinforced concrete SVR, FA Cement, coarse/fine aggregate, superplasticiser, the volume fraction of steel fibre, the length, diameter of steel fibre Uniaxial compressive strength, flexural strength Uniaxial compressive strength, flexural strength, cost R, MAE, RMSE, MAPE 299/
2692020 Huang et al[87] Strain-hardening cementitious composites AdaBoost, SVM, XGBoost, NSGA-III, UNSGA-III, ANN, CART Cement/fly ash/slag/rice husk/limestone/metakaolin/silica fume/sand/water -to-binder ratio, superplasticizer, fiber volume/length/diameter, fiber Young’s modulus Compressive strength, tensile strength, ductility Compressive strength, tensile strength, ductility, cost, CO2 emission R2, ME, MAE, MSE, MAD 745 2021 Mahjoubi et al[88] Rubbercrete M5 P, MGEP, GWO cement, water, coarse/fine aggregate, silica fume, superplasticizer, waste coarse rubber, waste fine rubber, concrete age Compressive strength Compressive strength, cost, CO2 emission, the amount waste rubber of consumption R, MAE, RMSE 712 2021 Golafshani et al[89] Electrically conductive cementitious composites SVR, BAS Age, cement, graphite powder, ground granulated blast furnace slag, steel slag Flexural strength, electrical conductivity Flexural strength, electrical conductivity, Cost R, MAPE, MAE, RMSE 252/
3362021 Sun et al[90] Graphite-based nanomaterials reinforced cementitious composites KNN, SVR, RFR, XGBoost, BPNN, BO, NSGA-II Cement, sand fitness modulus, sand, Graphite-based nanomaterials material/diameter/thickness/surface area of graphite-based nanomaterials, water, superplasticizer, ultrasonication, curing age, dry Uniaxial compressive strength, electrical resistivity Uniaxial compressive strength, electrical resistivity R2, MAE, MSE 379/416 2022 Dong et al[4] Green concrete MLR, KNN, SVM, GP, RF, ANN, GBM, XGBM Waste granite powder, waste marble powder, ground granulated blast furnace slag, fly ash, silica fume, cement, water, fine/coarse aggregates, superplasticizer, age Compressive Strength Compressive Strength, cost, global warming potential, acidification potential, fossil
fuel depletion potentialMSE, MAPE, MBE, RMSE, MAE, R2 2644 2023 Shamsabadi et al[91] Notes: MR - Multiple regression, GBM - Gradient boosting machine, XGBM - Extreme gradient boosting machine, SSE – Sum of the squares error, MRE – Mean relative error, ME – Maximum error, MBE – Mean bias error. -
[1] DE ROUSSEAU M A, KASPRZYK J R, SRUBAR III W V. Computational design optimization of concrete mixtures: a review[J]. Cement and Concrete Research, 2018, 109: 42-53. doi: 10.1016/j.cemconres.2018.04.007 [2] RUSSELL S, NORVIG P. Artificial Intelligence: A Modern Approach[M]. Prentice-Hall Inc, New Jersey, USA, 1995. [3] MARSLAND S. Machine Learning: An Algorithm Perspective[M]. Taylor & Francis, LLC, Florida, USA, 2015. [4] DONG W, HUANG Y M, LEHANE B, et al. Multi-objective design optimization for graphite-based nanomaterials reinforced cementitious composites: a data-driven method with machine learning and NSGA-II[J]. Construction and Building Materials, 2022, 331: 127198. doi: 10.1016/j.conbuildmat.2022.127198 [5] GUNAYDIN O, AKBAS E, OZBEYAZ A, et al. Machine learning based evaluation of concrete strength from saturated to dry by non-destructive methods[J]. Journal of Building Engineering, 2023, 76: 107174. doi: 10.1016/j.jobe.2023.107174 [6] ALKAYEM N F, SHEN L, MAYYA A, et al. Prediction of concrete and FRC properties at high temperature using machine and deep learning A review of recent advances and future perspectives[J]. Journal of Building Engineering, 2024, 83: 108369. doi: 10.1016/j.jobe.2023.108369 [7] WANG S Q, XIA P, CHEN K Y, et al. Prediction and optimization model of sustainable concrete properties using machine learning, deep learning and swarm intelligence A review[J]. Journal of Building Engineering, 2023, 80: 108065. doi: 10.1016/j.jobe.2023.108065 [8] NASERI H. Cost optimization of no-slump concrete using genetic algorithm and particle swarm optimization[J]. International Journal of Innovation, Management and Technology, 2019, 10. [9] KENNEDY J, EBERHART R. Particle swarm optimization[C]. In: 1995 IEEE International Conference on Neural Networks, 1995, pp. 1942-1948. [10] JIANG X, LI S. BAS: beetle antennae search algorithm for optimization problems. arXiv preprint, 2017, arXiv: 1710.10724. [11] ZHANG J F, HUANG Y M, MA G W, et al. Mixture optimization for environmental, economical and mechanical objectives in silica fume concrete a novel framework based on machine learning and a new meta-heuristic algorithm[J]. Resources, Conservation & Recycling, 2021, 167: 105395. [12] SONG Y, WANG X, LI H, et al. Mixture Optimization of Cementitious Materials Using Machine Learning and Metaheuristic Algorithms: State of the Art and Future Prospects[J]. Materials, 2022, 15(21): 7830. doi: 10.3390/ma15217830 [13] ZHOU C, STERKENS W, DÍAZ-ROMERO D J, et al. You Only Demanufacture once (YODO): WEEE retrieval using unsupervised learning[J]. Resources, Conservation & Recycling, 2023, 190: 106826. [14] RENARD S, CORBETT B, SWEI O. Minimizing the global warming impact of pavement infrastructure through reinforcement learning[J]. Resources, Conservation & Recycling, 2021, 167: 105240. [15] SUN R, SEVILLANO E, PERERA R. Debonding detection of FRP strengthened concrete beams by using impedance measurements and an ensemble PSO adaptive spectral model[J]. Composite Structures, 2015, 125: 374-387. doi: 10.1016/j.compstruct.2015.02.011 [16] LU W, YUAN L, XUE F. Investigating the bulk density of construction waste: a big data-driven approach[J]. Resources, Conservation & Recycling, 2021, 169: 105480. [17] CHEN W, XU J, DONG M, et al. Data-driven analysis on ultimate axial strain of FRP-confined concrete cylinders based on explicit and implicit algorithms[J]. Composite Structures, 2021, 268: 113904. doi: 10.1016/j.compstruct.2021.113904 [18] NIE Y, SHI J, HE Z, et al. Evaluation of high-volume fly ash (HVFA) concrete modified by metakaolin: technical, economic and environmental analysis[J]. Powder Technology, 2022, 397: 117121. doi: 10.1016/j.powtec.2022.117121 [19] ZHANG M, ZHANG C, ZHANG J, et al. Effect of composition and curing on alkali activated fly ash-slag binders: machine learning prediction with a random forest-genetic algorithm hybrid model[J]. Construction and Building Materials, 2023, 366: 129940. doi: 10.1016/j.conbuildmat.2022.129940 [20] BEHNOOD A, GOLAFSHANI E M. Artificial Intelligence to Model the Performance of Concrete Mixtures and Elements: A Review[J]. Archives of Computational Methods in Engineering, 2022, 29: 1941-1964. doi: 10.1007/s11831-021-09644-0 [21] 刘国华, 陈斌, 汪树玉等. 基于人工神经网络和Monte-Carlo法的混凝土配合比优化设计研究[J]. 水力发电学报, 2003, (4): 45-53. doi: 10.3969/j.issn.1003-1243.2003.04.007LIU Guohua, CHEN Bin, WANG Shuyu, et al. Optimization of concrete mixture on the basis of artificial neural network and Monte-Carlo techniques[J]. JOURNAL OF HYDROELECTRIC ENGINEERING, 2003, (4): 45-53(in Chinese). doi: 10.3969/j.issn.1003-1243.2003.04.007 [22] 李青云, 张建红, 包承纲. 风化花岗岩开挖弃料配制三峡二期围堰防渗墙材料[J]. 水利学报, 2004, (11): 114-118. doi: 10.3321/j.issn:0559-9350.2004.11.019LI Qingyun, ZHANG Jianhong, BAO Chenggang. Plastic concrete for cutoff wall using weathered granite sand in stage II cofferdam of Three Gorges Project[J]. SHUILI XUEBAO, 2004, (11): 114-118(in Chinese). doi: 10.3321/j.issn:0559-9350.2004.11.019 [23] JI T, LIN T W, LIN X J. A concrete mix proportion design algorithm based on artificial neural networks[J]. Cement and Concrete Research, 2006, 36: 1399-408. doi: 10.1016/j.cemconres.2006.01.009 [24] 陈晓东, 陈斌, 刘国华. 基于BP ANN-GA混合型算法的混凝土配合比优化设计研究[J]. 水力发电学报, 2007, (5): 59-63+52. doi: 10.3969/j.issn.1003-1243.2007.05.012CHEN Xiaodong, CHEN Bin, LIU Guohua. Optimization of concrete mixture based on BP ANN and genetic algorithms[J]. JOURNAL OF HYDROELECTRIC ENGINEERING, 2007, (5): 59-63+52(in Chinese). doi: 10.3969/j.issn.1003-1243.2007.05.012 [25] I-CHENG Yeh. Computer-aided design for optimum concrete mixtures[J]. Cement & Concrete Composites, 2007, 29: 193-202. [26] CHANDWANI V, AGRAWAL V, NAGAR R. Modeling slump of ready mix concrete using genetic algorithms assisted training of Artificial Neural Networks[J]. Expert Systems with Applications, 2015, 42: 885-93. doi: 10.1016/j.eswa.2014.08.048 [27] GOLAFSHANI E M, BEHNOOD A. Estimating the optimal mix design of silica fume concrete using biogeography-based programming[J]. Cement and Concrete Composites, 2019, 96: 95-105. doi: 10.1016/j.cemconcomp.2018.11.005 [28] ZIOLKOWSKI P, NIEDOSTATKIEWICZ M, KANG S B. Model-based adaptive machine learning approach in concrete mix design[J]. Materials, 2021, 14(7): 1661. doi: 10.3390/ma14071661 [29] LUNDBERG S M, LEE S I. A unified approach to interpreting model predictions[C]∥Advances in Neural Information Processing Systems. Long Beach, CA: NIPS, 2017, pp. 4765-4774. [30] NASER M Z. Digital twin for next gen concretes: on-demand tuning of vulnerable mixtures through explainable and anomalous machine learning[J]. Cement and Concrete Composites, 2022, 132: 104640. doi: 10.1016/j.cemconcomp.2022.104640 [31] MAHJOUBI S, BARHEMAT R, MENG W N, et al. Deep learning from physicochemical information of concrete with an artificial language for property prediction and reaction discovery[J]. Resources, Conservation and Recycling, 2023, 190: 106870. doi: 10.1016/j.resconrec.2023.106870 [32] YOUNG B A, HALL A, PILON L, et al. Can the compressive strength of concrete be estimated from knowledge of the mixture proportions ?: New insights from statistical analysis and machine learning methods[J]. Cement and Concrete Research, 2019, 115: 379-88. doi: 10.1016/j.cemconres.2018.09.006 [33] I-CHENG Yeh. Design of high-performance concrete mixture using neural networks and nonlinear programming[J]. Journal of Computing in Civil Engineering, 1999, 13: 36-42. doi: 10.1061/(ASCE)0887-3801(1999)13:1(36) [34] NASERI H, JAHANBAKHSH H, HOSSEINI P, et al. Designing sustainable concrete mixture by developing a new machine learning technique[J]. Journal of Cleaner Production, 2020, 258: 120578. doi: 10.1016/j.jclepro.2020.120578 [35] ZHU F, WU X P, ZHOU M M, et al. Intelligent design of building materials: development of an ai-based method for cement-slag concrete design[J]. Materials, 2022, 15(11): 3833. doi: 10.3390/ma15113833 [36] NASERI H, HOSSEINI P, JAHANBAKHSH H, et al. A novel evolutionary learning to prepare sustainable concrete mixtures with supplementary cementitious materials[J]. Environment, Development and Sustainability 2023, 25: 5831-5865. [37] ZHENG W, SHUI Z H, XU Z Z, et al. Multi-objective optimization of concrete mix design based on machine learning[J]. Journal of Building Engineering, 2023, 76: 107396. doi: 10.1016/j.jobe.2023.107396 [38] LIM C H, YOON Y S, KIM J H. Genetic algorithm in mix proportioning of high-performance concrete[J]. Cement and Concrete Research, 2004, 34: 409-420. doi: 10.1016/j.cemconres.2003.08.018 [39] JAYARAM M A, NATARAJA M C, RAVIKUMAR C N. Elitist genetic algorithm models optimization of high performance concrete mixes[J]. Materials and Manufacturing Processes, 2009, 24: 225-229. doi: 10.1080/10426910802612387 [40] LEE J H, YOON Y S, KIM J H. A new heuristic algorithm for mix design of high performance concrete[J]. KSCE Journal of Civil Engineering, 2012, 16(6): 974-979. doi: 10.1007/s12205-012-1011-0 [41] CHENG M Y, PRAYOGO D, WU Y W. Novel genetic algorithm-based evolutionary support vector machine for optimizing high-performance concrete mixture[J]. Journal of Computing in Civil Engineering, 2014, 28(4): 06014003. doi: 10.1061/(ASCE)CP.1943-5487.0000347 [42] KE X Y, DUAN Y. A Bayesian machine learning approach for inverse prediction of high-performance concrete ingredients with targeted performance[J]. Construction and Building Materials, 2021, 270: 121424. doi: 10.1016/j.conbuildmat.2020.121424 [43] CHENG M Y, CAO M T, DAO-THI N M. A novel artificial intelligence-aided system to mine historical high-performance concrete data for optimizing mixture design[J]. Expert Systems with Applications, 2023, 212: 118605. doi: 10.1016/j.eswa.2022.118605 [44] 陈庆, 马瑞, 蒋正武等. 基于GA-BP神经网络的UHPC抗压强度预测与配合比设计[J]. 建筑材料学报, 2020, 23(1): 176-183+191. doi: 10.3969/j.issn.1007-9629.2020.01.027CHEN Qing, MA Rui, JIANG Zhengwu, et al. Compressive strength prediction and mix proportion design of UHPC based on GA-BP neural network[J]. JOURNAL OF BUILDING MATERIALS, 2020, 23(1): 176-183+191(in Chinese). doi: 10.3969/j.issn.1007-9629.2020.01.027 [45] FAN D Q, YU R, SHUI Z H, et al. A novel approach for developing a green ultra-high performance concrete (UHPC) with advanced particles packing meso-structure[J]. Construction and Building Materials, 2020, 265: 120339. doi: 10.1016/j.conbuildmat.2020.120339 [46] FAN D Q, YU R, FU S Y, et al. Precise design and characteristics prediction of ultra-high performance concrete (UHPC) based on artificial intelligence techniques[J]. Cement and Concrete Composites, 2021, 122: 104171. doi: 10.1016/j.cemconcomp.2021.104171 [47] ZOU D L, WU L L, HAO Y F, et al. Composition-strength relationship study of ultrahigh performance fiber reinforced concrete (UHPFRC) using an interpretable data-driven approach.[J]. Construction and Building Materials, 2023, 392: 131973. doi: 10.1016/j.conbuildmat.2023.131973 [48] ABELLAN-GARCIA J, FERNÁNDEZ J, KHAN M I, et al. Uniaxial tensile ductility behavior of ultrahigh-performance concrete based on the mixture design - Partial dependence approach[J]. Cement and Concrete Composites, 2023, 140: 105060. doi: 10.1016/j.cemconcomp.2023.105060 [49] 王海超, 何世钦. 人工神经网络技术在高强混凝土中的应用——配合比设计初探[J]. 建筑材料学报, 2004, (1): 94-101. doi: 10.3969/j.issn.1007-9629.2004.01.018WANG Haichao, HE Shiqin. Application of artificial neural network in the design of high performance concrete——primary study on mix proportion[J]. JOURNAL OF BUILDING MATERIALS, 2004, (1): 94-101(in Chinese). doi: 10.3969/j.issn.1007-9629.2004.01.018 [50] LI Y, LI H W, LI Y N, et al. Optimum design of high strength concrete mix proportion for crack resistance using artificial neural networks and genetic algorithm[J]. Frontiers in Materials, 2020, 7: 590661. doi: 10.3389/fmats.2020.590661 [51] ZHANG J F, HUANG Y M, WANG Y H, et al. Multi-objective optimization of concrete mixture proportions using machine learning and metaheuristic algorithms[J]. Construction and Building Materials, 2020, 253: 119208. doi: 10.1016/j.conbuildmat.2020.119208 [52] 吴贤国, 王雷, 陈虹宇等. 基于随机森林-NSGA Ⅱ高性能混凝土耐久性配合比的多目标优化研究[J]. 材料导报, 2022, 36(17): 115-121.WU Xianguo, WANG Lei, CHEN Hongyu, et al. Multi-objective optimization of high-performance concrete durability mix ratio based on RF-NSGA II[J]. MATERIALS REPORTS, 2022, 36(17): 115-121(in Chinese). [53] 任秋兵, 李文伟, 李明超等. 水工高性能混凝土配合比多目标智能优化设计与分析方法[J]. 水利学报, 2022, 53(1): 98-108.REN Qiubing, LI Wenwei, LI Mingchao, et al. Multi-objective intelligent optimization design and analysis method for mix proportion of hydraulic high performance concrete[J]. SHUILI XUEBAO, 2022, 53(1): 98-108(in Chinese). [54] CHEN B, WANG L, FENG Z B, et al. Optimization of high-performance concrete mix ratio design using machine learning[J]. Engineering Applications of Artificial Intelligence, 2023, 122: 106047. doi: 10.1016/j.engappai.2023.106047 [55] YANG S, CHEN H Y, FENG Z B, et al. Intelligent multi-objective optimization for high-performance concrete mix proportion design A hybrid machine learning approach[J]. Engineering Applications of Artificial Intelligence, 2023, 126: 106868. doi: 10.1016/j.engappai.2023.106868 [56] SADROSSADAT E, BASARIR H, KARRECH A, et al. Multi-objective mixture design and optimization of steel fiber reinforced UHPC using machine learning algorithms and metaheuristics[J]. Engineering with Computers, 2022, 38(S3): S2569-2582. doi: 10.1007/s00366-021-01403-w [57] SALEH E, TARAWNEH A, NASER M Z, et al. You only design once (YODO): Gaussian process-batch bayesian optimization framework for mixture design of ultra high performance concrete[J]. Construction and Building Materials, 2022, 330: 127270. doi: 10.1016/j.conbuildmat.2022.127270 [58] MAHJOUBI S, BARHEMAT R, MENG W N, et al. AI-guided auto-discovery of low-carbon cost-effective ultra-high performance concrete (UHPC)[J]. Resources, Conservation and Recycling, 2023, 189: 106741. doi: 10.1016/j.resconrec.2022.106741 [59] TIPU R K, PANCHAL V R, PANDYA K S. Multi-objective optimized high-strength concrete mix design using a hybrid machine learning and metaheuristic algorithm[J]. Asian Journal of Civil Engineering, 2023, 24: 849-867. doi: 10.1007/s42107-022-00535-8 [60] PARK W. Genetic-algorithm-based mix proportion design method for recycled aggregate concrete[J]. Transactions of the Canadian Society for Mechanical Engineering, 2013, 37. [61] ŠIPOŠ T K, MILICEVIC I, SIDDIQUE R. Model for mix design of brick aggregate concrete based on neural network modelling[J]. Construction and Building Materials, 2017, 148: 757-769. doi: 10.1016/j.conbuildmat.2017.05.111 [62] REZAIEE-PAJAND M, ABAD J M N, KARIMIPOUR A, et al. Propose new implement models to determine the compressive, tensile and flexural strengths of recycled coarse aggregate concrete via imperialist competitive algorithm[J]. Journal of Building Engineering 40 (2021) 2021, 40: 102337. [63] NUNEZ I, MARANI A, NEHDI M L. Mixture optimization of recycled aggregate concrete using hybrid machine learning model[J]. Materials, 2020, 13: 4331. doi: 10.3390/ma13194331 [64] ZHANG J F, HUANG Y M, ASLANI F, et al. A hybrid intelligent system for designing optimal proportions of recycled aggregate concrete[J]. Journal of Cleaner Production, 2020, 273: 122922. doi: 10.1016/j.jclepro.2020.122922 [65] WANG S Q, XIA P, WANG Z, et al. Intelligent mix design of recycled brick aggregate concrete based on swarm intelligence[J]. Journal of Building Engineering, 2023, 71: 106508. doi: 10.1016/j.jobe.2023.106508 [66] 刘凯华, 郑佳凯, 谢维力等. 基于机器学习的再生混凝土配合比设计方法[J]. 湖南大学学报(自然科学版), 2023, 50(9): 88-96.LIU Kaihua, ZHENG Jiakai, XIE Weili, et al. Mixture design method of recycled aggregate concrete based on machine learning[J]. Journal of Hunan University (Natural Sciences), 2023, 50(9): 88-96(in Chinese). [67] LIU K H, ZHENG J K, DONG S X, et al. Mixture optimization of mechanical, economical, and environmental objectives for sustainable recycled aggregate concrete based on machine learning and metaheuristic algorithms[J]. Journal of Building Engineering, 2023, 63: 105570. doi: 10.1016/j.jobe.2022.105570 [68] LOKUGE W, WILSON A, GUNASEKARA C, et al. Design of fly ash geopolymer concrete mix proportions using Multivariate Adaptive Regression Spline model[J]. Construction and Building Materials, 2018, 166: 472-481. doi: 10.1016/j.conbuildmat.2018.01.175 [69] GUNASEKARA C, ATZARAKIS P, LOKUGE W, et al. Novel analytical method for mix design and performance prediction of high calcium fly ash geopolymer concrete[J]. Polymers, 2021, 13: 900. doi: 10.3390/polym13060900 [70] SUN Y B, CHENG H, ZHANG S Z, et al. Prediction & optimization of alkali-activated concrete based on the random forest machine learning algorithm[J]. Construction and Building Materials, 2023, 385: 131519. doi: 10.1016/j.conbuildmat.2023.131519 [71] SHOBEIRI V, BENNETT B, XIE T Y, et al. A generic framework for augmented concrete mix design: optimization of geopolymer concrete considering environmental, financial and mechanical properties[J]. Journal of Cleaner Production, 2022, 369: 133382. doi: 10.1016/j.jclepro.2022.133382 [72] DONG W, HUANG Y M, CUI A D, et al. Mix design optimization for fly ash-based geopolymer with mechanical, environmental, and economic objectives using soft computing technology[J]. Journal of Building Engineering, 2023, 72: 106577. doi: 10.1016/j.jobe.2023.106577 [73] HUANG Y M, HUO Z H, MA G W, et al. Multi-objective optimization of fly ash-slag based geopolymer considering strength, cost and CO2 emission: A new framework based on tree-based ensemble models and NSGA-II[J]. Journal of Building Engineering, 2023, 68: 106070. doi: 10.1016/j.jobe.2023.106070 [74] LI Y, SHEN J L, LIN H, et al. Optimization design for alkali-activated slag-fly ash geopolymer concrete based on artificial intelligence considering compressive strength, cost, and carbon emission.[J]. Journal of Building Engineering, 2023, 75: 106929. doi: 10.1016/j.jobe.2023.106929 [75] SIFAN M, NGUYEN H, NAGARATNAM B, et al. Efficient mix design method for lightweight high strength concrete: A machine learning approach[J]. Structures, 2023, 55: 1805-1822. doi: 10.1016/j.istruc.2023.06.122 [76] HUSSAIN F, KHAN S A, KHUSHNOOD R A, et al. Machine learning-based predictive modeling of sustainable lightweight aggregate concrete[J]. Sustainability, 2023, 15: 641. [77] DABBAGHI F, TANHADOUST A, NEHDI M L, et al. Life cycle assessment multi-objective optimization and deep belief network model for sustainable lightweight aggregate concrete[J]. Journal of Cleaner Production, 2021, 318: 128554. doi: 10.1016/j.jclepro.2021.128554 [78] ZHANG J F, HUANG Y M, MA G W, et al. Automating the mixture design of lightweight foamed concrete using multi-objective firefly algorithm and support vector regression[J]. Cement and Concrete Composites, 2021, 121: 104103. doi: 10.1016/j.cemconcomp.2021.104103 [79] KAO C Y, SHEN C H, JAN J C, et al. A computer-aided approach to pozzolanic concrete mix design[J]. Advances in Civil Engineering, 2018: 4398017. [80] SADROSSADAT E, BASARIR H, LUO G H, et al. Multi-objective mixture design of cemented paste backfill using particle swarm optimization algorithm[J]. Minerals Engineering, 2020, 153: 106385. doi: 10.1016/j.mineng.2020.106385 [81] 韩斌, 吉坤, 胡亚飞等. ANN-PSO-GA模型在湿喷混凝土强度预测及配合比优化中的应用[J]. 采矿与安全工程学报, 2021, 38(3): 584-591.HAN Bin, JI Kun, HU Yafei, et al. Application of ANN-PSO-GA model in UCS prediction and mix proportion optimization of wet shotcrete[J]. Journal of Mining & Safety Engineering, 2021, 38(3): 584-591(in Chinese). [82] REN J L, ZHAO H B, ZHANG L, et al. Design optimization of cement grouting material based on adaptive boosting algorithm and simplicial homology global optimization[J]. Journal of Building Engineering, 2022, 49: 104049. doi: 10.1016/j.jobe.2022.104049 [83] PENIDO R E K, PAIXAO R C F D, COSTA L C B, et al. Predicting the compressive strength of steelmaking slag concrete with machine learning - Considerations on developing a mix design tool[J]. Construction and Building Materials, 2022, 341: 127896. doi: 10.1016/j.conbuildmat.2022.127896 [84] ZHAO W J, FENG S Y, LIU J X, et al. An explainable intelligent algorithm for the multiple performance prediction of cement-based grouting materials[J]. Construction and Building Materials, 2023, 366: 130146. doi: 10.1016/j.conbuildmat.2022.130146 [85] HAN F L, LV Y, LIU Y, et al. Exploring interpretable ensemble learning to predict mechanical strength and thermal conductivity of aerogel incorporated concrete[J]. Construction and Building Materials, 2023, 392: 131781. doi: 10.1016/j.conbuildmat.2023.131781 [86] ZHANG J F, HUANG Y M, MA G W, et al. A metaheuristic-optimized multi-output model for predicting multiple properties of pervious concrete[J]. Construction and Building Materials, 2020, 249: 118803. doi: 10.1016/j.conbuildmat.2020.118803 [87] HUANG Y M, ZHANG J F, ANN F T, et al. Intelligent mixture design of steel fiber reinforced concrete using a support vector regression and firefly algorithm based multi-objective optimization model[J]. Construction and Building Materials, 2020, 260: 120457. doi: 10.1016/j.conbuildmat.2020.120457 [88] MAHJOUBI S, BARHEMAT R, GUO P W, et al. Prediction and multi-objective optimization of mechanical, economical, and environmental properties for strain-hardening cementitious composites (SHCC) based on automated machine learning and metaheuristic algorithms[J]. Journal of Cleaner Production, 2021, 329: 129665. doi: 10.1016/j.jclepro.2021.129665 [89] GOLAFSHANI E M, ARASHPOUR M, KASHANI A. Green mix design of rubber concrete using machine learning-based ensemble model and constrained multi-objective optimization[J]. Journal of Cleaner Production, 2021, 327: 129518. doi: 10.1016/j.jclepro.2021.129518 [90] SUN J B, WANG X Y, ZHANG J F, et al. Multi-objective optimization of a graphite-slag conductive composite applying a BAS-SVR based model[J]. Journal of Building Engineering, 2021, 44: 103223. doi: 10.1016/j.jobe.2021.103223 [91] SHAMSABADI E A, SALEHPOUR M, ZANDIFAEZ P, et al. Data-driven multicollinearity-aware multi-objective optimization of green concrete mixes[J]. Journal of Cleaner Production, 2023, 390: 136103. doi: 10.1016/j.jclepro.2023.136103 [92] LI Z, YOON J, ZHANG R, et al. Machine learning in concrete science: applications, challenges, and best practices[J]. npj Computational Materials, 2022, 8(1): 127. doi: 10.1038/s41524-022-00810-x -
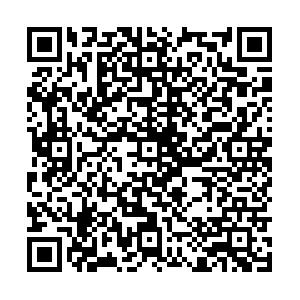
计量
- 文章访问数: 70
- HTML全文浏览量: 72
- 被引次数: 0