Fatigue assessment for composites by using piezoelectric signal
-
摘要: 复合材料结构应变特征随着疲劳加载发生变化,因此本文尝试实时监测疲劳周期内复合材料结构的应变特征信号,通过应变信号评估结构疲劳寿命。然而已有研究表明,电阻式应变片在长时间动态测试中经常出现提前疲劳失效,不适合疲劳全周期内应变信号采集。为此,具有高耐疲劳性的新型聚偏氟乙烯压电薄膜(PVDF)被用于采集复合材料结构疲劳特征信号。通过在碳纤维增强树脂基复合材料(T700/9A16)层合板表面粘贴PVDF薄膜,获取层合板疲劳过程中的压电信号。基于压电效应,将表征疲劳损伤状态的应变信息转化为PVDF压电信号输出。基于试验生成的压电信号数据库,采用随机森林回归算法高效地建立压电信号与复合材料层合板疲劳循环次数之间的关联。通过不同循环次数下的PVDF压电信号可以准确预测试验件的实际疲劳加载次数,实现了对复合材料结构疲劳状态的有效评估。Abstract: Considering the strain characteristics of the composite structures changing with fatigue loading, so that this paper attempts to monitor the strain characteristics in real time during the fatigue cycle and assesses the fatigue life through the strain signals. However, from the published literatures, resistance strain gauge often suffered from early fatigue failure in the long-time dynamic testing, which is not suitable for strain signal acquisition during the full fatigue cycle. Therefore, the novel polyvinylidene fluoride piezoelectric film (PVDF) with high fatigue resistance is used to acquire fatigue characteristic signals of composite structures. The piezoelectric signals during the fatigue process of the composite laminates are obtained by pasting PVDF on the surface of carbon fiber reinforced plastic (CFRP) plates (T700/9A16). Based on the piezoelectric effect, the strain information during the fatigue process is converted into the piezoelectric signal from PVDF. Then, a Random Forest Regression (RFR) algorithm is trained based on the database generated from the experimental tests to efficiently establish the correlation between the piezoelectric signals and fatigue cycles of composite laminates. Through the trained RFR network, the actual fatigue cycles of the test pieces can be accurately predicted based on the piezoelectric signals, in which the maximum percentage error of logarithms of fatigue cycles is controlled within 5%. This paper provides a new research idea and technical support for the fatigue life assessment of composite materials.
-
Key words:
- Composite plate /
- residual stiffness /
- Fatigue /
- PVDF /
- Fatigue assessment /
- Random Forest Regression
-
算法I. 随机森林回归算法 输入:训练数据集DNum,由当前压电信号Vi及对应循环次数ni构成。 输出:预测的当前循环周次npredict 。 Step1: For i = 1 to Ntree (a) 从初始训练集DNum中随机且有放回地抽取训练样本z*;(b) 基于抽取样本z*开展决策树训练。训练决策树模型节点时,在所有样本特征中随机选取部分特征,确定最优切分变量与切分点[26],完成最优决策树训练。 Step2: 基于Step1,完成所有最优决策树训练${\{ {T_{best}}\} ^{{N_{tree}}}} $。 Step3: 输入任意的电压信号Vt,输出疲劳循环周次预测结果$ {n_{predict}}({V_t}) $ = $ \sum\nolimits_{i = 1}^{{N_{tree}}} {{T_{best}}} ({V_t})/{N_{tree}} $。 表 1 碳纤维复合材料(T700/9A16)基本材料参数
Table 1. Material properties of the CFRP (T700/9A16)
Material properties E11/GPa 126.97 E22/GPa 8.52 G12/GPa 3.41 XT/MPa 2433.05 XC/MPa 1063.25 YT/MPa 37.85 YC/MPa 118.30 S12/MPa 61.30 Notes: E11 and E22 represent the tensile moduli in the fiber and matrix directions, respectively. G12 denotes the shear modulus. XT and YT represent tensile strength in the fiber and matrix directions. XC and YC represent compression strength in the fiber and matrix directions. S12 denotes the shear strength. 表 2 碳纤维复合材料(T700/9A16)疲劳试验件疲劳寿命
Table 2. Fatigue life of the carbon fiber reinforced plastic laminates (T700/9A16)
Test piece number Fatigue life Logarithmic fatigue life PL -1 9302 3.97 PL -2 25642 4.41 PL-3 26324 4.42 PL-4 - - PL-5 20175 4.30 PL-6 9715 3.99 Mean 18231.6 4.22 SD 8313.36 0.22 CoV 0.46 0.05 Notes: SD represents Standard Deviation and CoV denotes Coefficient of Variation. 表 2 加载n次疲劳载荷后的碳纤维复合材料(T700/9A16)试验件剩余刚度以及剩余强度
Table 2. Residual stiffness and residual strength of CFRP (T700/9A16) test pieces after n number cycles of fatigue loading
Test piece number Cyclic number (n) Ultimate load/N Residual strength/MPa Residual stiffness/GPa PLE-0-1 0 9646.8 643.1 34.48 PLE-0-2 0 10461.7 697.4 33.45 PLE-2000-1 2000 8806.4 587.1 21.77 PLE-2000-2 2000 9750.8 650.1 19.26 PLE-5000-1 5000 8773.8 584.9 21.77 PLE-5000-2 5000 9807.1 653.8 20.41 PLE-10000-1 10000 8336 555.7 19.08 PLE-10000-2 10000 9253.3 616.9 16.28 PLE-15000-1 15000 8964 597.6 11.29 Notes: The test piece number is defined as PLE-X-Y, in which PLE represents the residual properties of fatigue test pieces, X denotes the number cycles of fatigue loading and Y denotes the test number. 表 4 含PVDF碳纤维复合材料(T700/9A16)试验件疲劳开始阶段与结束阶段输出的压电信号峰值平均值
Table 4. Average peak value of piezoelectric signals at starting /ending stage for CFRP (T700/9A16) test pieces with PVDF
Test piece number Average peak voltage at starting stage /V Average peak voltage at ending stage /V PL-PVDF-1 3.02 3.49 PL-PVDF-2 2.63 2.76 PL-PVDF-3 3.09 3.23 PL-PVDF-4 3.63 3.80 PL-PVDF-5 3.05 3.42 PL-PVDF-6 3.17 3.52 PL-PVDF-7 3.07 3.56 PL-PVDF-8 3.08 3.48 PL-PVDF-9 2.68 2.84 PL-PVDF-10 2.84 3.12 PL-PVDF-11 3.08 3.47 PL-PVDF-12 3.56 3.88 PL-PVDF-13 3.11 3.52 PL-PVDF-14 3.04 3.48 Notes: The test piece number is defined as PL-PVDF-NN, in which PL represents fatigue test pieces, PVDF denotes the polyvinylidene fluoride piezoelectric film and NN denotes the test number. 表 5 随机森林算法中超参数选取值
Table 5. The selected values for hyperparameters in RFR
NumTrees Method MinLeafSize Surrogate 300 Regression 5 on 表 6 数据驱动算法误差指标对比
Table 6. Comparison of error indicators for different models
Data-driven algorithm MAE MSE R-square RFR 0.0946 0.0200 0.9201 SVM 0.1315 0.0292 0.8845 XGBoost 0.0761 0.0065 0.9461 BPNN 0.3347 0.0672 0.8281 Notes: RFR denotes Random Forest Regression; SVM represents Support Vector Machine; XGBoost denotes EXtreme Gradient Boosting and BPNN represents Back propagation neural network. MAE, MSE and R-square denote the Mean Absolute Error, Mean Square Error and Coefficient of determination, respectively. 表 7 基于压电信号的碳纤维复合材料试验件(T700/9A16)疲劳循环周次及剩余寿命估计结果
Table 7. The fatigue cycles and remaining life of CFRP test pieces (T700/9A16) predicted by using RFR
Test piece number $ {n_{test}} $ ${n_{pre}}$ Error ${\tilde n_{test}}$ ${\tilde n_{pre}}$ Error Piece 1 3.30 3.24 1.93% 4.39 4.21 4.10% Piece 2 3.70 3.86 4.48% 4.18 4.03 3.59% Piece 3 4.00 4.13 3.27% 3.92 3.65 6.89% Notes: $ {n_{test}} $ denotes the logarithms of tested cycling number; ${n_{pre}}$ denotes the logarithms of predicted cycling number by using trained RFR; ${\tilde n_{test}}$ represents the tested remaining logarithmic fatigue life; ${\tilde n_{pre}}$ represents the predicted remaining logarithmic fatigue life. -
[1] AHMED O, WANG X, TRAN MV, et al. Advancements in fiber-reinforced polymer composite materials damage detection methods: Towards achieving energy-efficient SHM systems[J]. Composite Part B Engineering, 2021, 223: 109136. doi: 10.1016/j.compositesb.2021.109136 [2] STASZEWSKI WJ, MAHZAN S, TRAYNOR R. Health monitoring of aerospace composite structures active and passive approach[J]. Composite Science & Technology, 2009, 69: 1678-1685. [3] PENG T, WANG S, HUANG YD, et al. Study of the fatigue behavior of the unidirectional Zylon/epoxy composite used in pulsed magnets[J]. IEEE Transactions on Applied Superconductivity, 2020, 30(4): 4300705. [4] JEBRI L, ABBASSI F, DEMIRAL M, et al. Experimental and numerical analysis of progressive damage and failure behavior of carbon Woven-PPS[J]. Composite Structures, 2020, 243: 112234. doi: 10.1016/j.compstruct.2020.112234 [5] 王瑞金. 应变片在动态测试中疲劳规律的研究[J]. 机电工程, 2000, 4: 66-68. doi: 10.3969/j.issn.1001-4551.2000.04.026WANG RJ. The study of the fatigue law in dynamic test[J]. Journal of Mechanical & Electrical Engineering, 2000, 4: 66-68(in Chinese). doi: 10.3969/j.issn.1001-4551.2000.04.026 [6] SHIN YH, JUNG I, PARK H, et al. Mechanical fatigue resistance of piezoelectric PVDF polymers[J]. Micromachines, 2018, 9: 503-513. doi: 10.3390/mi9100503 [7] SANTE Di R. Fiber optic sensors for structural health monitoring of aircraft composite structures: Recent advances and applications[J]. Sensors, 2015, 15: 18666-18713, doi: 10.3390/s150818666 [8] DU X, ZHOU H, SUN W, et al. Graphene/epoxy interleaves for delamination toughening and monitoring of crack damage in carbon fibre/epoxy composite laminates[J]. Composite Science & Technology, 2017, 140: 123-133, [9] ANDREADES C, MAHMOODI P, CIAMPA F. Characterization of smart CFRP composites with embedded PZT transducers for nonlinear ultrasonic applications[J]. Composite Structures, 2018, 206: 456-466, doi: 10.1016/j.compstruct.2018.08.083 [10] AKAY E, YILMAZ C, KOCAMAN SE, et al. Monitoring Poisson’s ratio degradation of FRP composites under fatigue loading using biaxially embedded FBG sensors[J]. Materials, 2016, 9: 781-796. doi: 10.3390/ma9090781 [11] BUGGISCH C, GIBHARDT D, FELMET N, et al. Strain sensing in GFRP via fully integrated carbon nanotube epoxy film sensors[J]. Composite Part C, 2021, 6: 100191, [12] MASMOUDI S, El MAHI A, TURKI S. Fatigue behaviour and structural health monitoring by acoustic emission of E-glass/epoxy laminates with piezoelectric implant[J]. Applied Acoustics, 2016, 108: 50-58. doi: 10.1016/j.apacoust.2015.10.024 [13] RAMAKRISHNAN M, RAJAN G, SEMENOVA Y, et al. Overview of fiber optic sensor technologies for strain/temperature sensing applications in composite materials[J]. Sensors, 2016, 16(1): 99-126. doi: 10.3390/s16010099 [14] FENG T, FERRI ALIABADI MH, Structural integrity assessment of composites plates with embedded PZT transducers for structural health monitoring[J]. Materials, 2021, 14(20): 6148. [15] GHAFARI E, LU N, Self-polarized electro spun polyvinylidene fluoride (PVDF) nanofiber for sensing applications[J]. Composites Part B Engineering, 2019, 160: 1-9. [16] YU H, HUANG T, LU M, et al. Enhanced power output of an electro spun PVDF/MWCNTs-based nanogenerator by tuning its conductivity[J]. Nanotechnology, 2013, 24: 405401. doi: 10.1088/0957-4484/24/40/405401 [17] GARCIA C, TRENDAFILOVA I. Triboelectric sensor as a dual system for impact monitoring and prediction of the damage in composite structures[J]. Nano Energy, 2019, 60: 527-535. doi: 10.1016/j.nanoen.2019.03.070 [18] KNAPP J, ALTMANN E, NIEMANN J, et al. Measurement of shock events by means of strain gauges and accelerometer[J]. Measurement, 1998, 24(2): 87-96. doi: 10.1016/S0263-2241(98)00036-0 [19] 李焰, 钟方平, 刘乾, 等. PVDF在动态应变测量中的应用[J]. 爆炸与冲击, 2003, 3: 230-234. doi: 10.3321/j.issn:1001-1455.2003.03.007LI Y, ZHONG FP, LIU Q, et al. Application of PVDF in dynamic strain measurement[J]. Explosion and Shock Waves, 2003, 3: 230-234(in Chinese). doi: 10.3321/j.issn:1001-1455.2003.03.007 [20] GLOWACZ GA. Fault diagnosis of electric impact drills using thermal imaging[J]. Measurement, 2021, 171: 108815. doi: 10.1016/j.measurement.2020.108815 [21] KOUZIOKAS GN. A method for multi-class sentiment classification based on an improved one-vs-one (OVO) strategy and the support vector machine (SVM) algorithm[J]. Information Sciences, 2017, 394: 38-52. [22] LI JK, LIN M. Ensemble learning with diversified base models for fault diagnosis in nuclear power plants[J]. Annals of Nuclear Energy, 2021, 158: 108265. doi: 10.1016/j.anucene.2021.108265 [23] 翟洪军, 姚卫星. 纤维增强树脂基复合材料的疲劳剩余刚度研究进展[J]. 力学进展, 2022, 32(1): 69-80.ZHAI HJ, YAO WX. A survey on stiffness reduction of fiber reinforced plastic under cyclic loading[J]. Advance in Mechanics, 2022, 32(1): 69-80 (in Chinese). [24] 廉伟, 姚卫星. 复合材料层合板剩余刚度-剩余强度关联模型[J]. 复合材料学报, 2008, 25(5): 151-156. doi: 10.3321/j.issn:1000-3851.2008.05.025LIAN W, YAO WX. Residua stiffness residual strength coupled model of composite laminates[J]. Acta Materia ae Compositae Sinica, 2008, 25(5): 151-156 (in Chinese). doi: 10.3321/j.issn:1000-3851.2008.05.025 [25] 白石, 周智, 申宇, 等. 基于PVDF的无线智能疲劳监测系统[J]. 航空学报, 2014, 35(08): 2190-2198.BAI S, ZHOU Z, SHEN Y, et al. A wireless intelligent fatigue monitoring system based on PVDF[J]. Acta Aeronautica et Astronautica Sinica 2014, 35(08): 2190-2198(in Chinese). [26] PRADEEP K, DARPE AK, KULKARNI MS. An ensemble decision tree methodology for remaining useful life prediction of spur gears under natural pitting progression[J]. Structural Health Monitoring, 2019, 9(3): 854-872. [27] ASTM D3479/D3479M-19. Standard Test Method for Tension-Tension Fatigue of Polymer Matrix Composite Materials [S]. West Conshohocken, PA: ASTM International, 2019. [28] ASTM D3039/D3039M-17. Standard Test Method for Tension Properties of Polymer Matrix Composite Materials [S]. West Conshohocken, PA: ASTM International, 2017. [29] KUMAR R, KUMAR A, KUMAR DR. Buckling response of CNT based hybrid FG plates using finite element method and machine learning method[J]. Composite Structures, 2023, 319: 117204. doi: 10.1016/j.compstruct.2023.117204 -
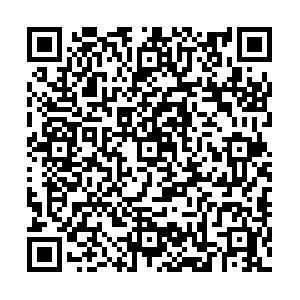
计量
- 文章访问数: 222
- HTML全文浏览量: 150
- 被引次数: 0