Artificial neural network-based mapping of microscopic damage to macroscopic stiffness in solid propellants
-
摘要: 作为一种高夹杂比颗粒增强聚合物基复合材料,固体推进剂的宏观力学性能主要由其细观结构决定。外加载荷下,初始缺陷或细观颗粒团聚均可诱发局部应力集中,导致颗粒-基体细观界面脱粘,材料宏观力学性能劣化。如何构建细观损伤与宏观性能间的映射关系,已成为推进剂细观实验结果合理运用、固体火箭发动机结构灾变准确预报的关键。为此,本文发展了基于连续介质力学框架的人工神经网络,以变形梯度的不变量为输入、自由能为输出,遴选现有自由能函数和损伤增长函数形式为神经网络设计激活函数,使神经网络先验地满足变形连续性、坐标不变性、热力学一致性等要求。基于上述物理相容性,神经网络能在稀疏训练数据条件下快速收敛,还能够自下而上地实现损伤状态的遗传映射。最后,采用有限元分析获取的数据集,验证了该网络模型对不同预损伤下的推进剂在单轴拉伸、等双轴拉伸、纯剪切三种加载条件下的宏观刚度预报能力。Abstract: As a particle-reinforced polymer composite with high inclusion ratio, the macro-mechanical properties of solid propellants depend on their meso-structures. Especially, under external loads, the stress concentration usually happens besides the regions of the initial imperfections and the particle agglomerations, leading to the interfacial debonding between the particles and the polymeric binders, consequently deteriorating macroscopic mechanical properties. How to build a relationship between the microscopic damage states and the macroscopic mechanical performances is the key issue for both the rational usage of the microscopic experimental results of solid propellants and the accurate prediction of structural disasters in solid rocket motors. For this purpose, this article develops an artificial neural network (ANN) based on the framework of continuum mechanics, with the scalar invariant of the deformation gradient tensor as the input and the scalar free energy as the output. Existing free energy functions and damage growth functions are selected as the activation functions of the ANN, and therefore the ANN can naturally satisfy the requirements of the continuum mechanics, including the deformation continuity, the coordinate invariance, and the thermodynamic consistency. These merits can guarantee the rapid convergence of the ANN with sparse training data, and additionally can obtain a bottom-up mapping of the microscopic damage states towards the macroscopic mechanical performances. Finally, using the dataset obtained from finite element analysis, the predictive ability of the ANN on the mechanical properties of solid propellants with different pre-damage states under uniaxial tension, biaxial tension, and pure shear are validated.
-
图 1 固体推进剂细观脱粘机理:(a)固体推进剂微观结构SEM图像;(b)无量纲裂纹长度$ \bar l = {\raise0.7 ex\hbox{$l$} \mathord{\left/ {\vphantom {l d}}\right.} \lower0.7 ex\hbox{$d$}} $[10]
Figure 1. Microscopic debonding mechanism of solid propellants: (a) SEM image of solid propellants microstructure; (b) Dimensionless crack length $ \bar l = {\raise0.7 ex\hbox{$l$} \mathord{\left/ {\vphantom {l d}}\right.} \lower0.7 ex\hbox{$d$}} $[10]
图 2 55 vol%颗粒增强粘合剂基体复合材料代表性体积元(RVE)模型及其在三种变形下的仿真应力云图,应力计量对基体剪切模量${\mu _{\text{m}}}$进行归一化
Figure 2. Geometry model and simulation stress clouds under three different deformations for the representative volume element (RVE) of 55 vol% particle reinforced binder matrix composite with stress metrology normalized to the matrix shear modulus ${\mu _{\text{m}}}$
图 3 数值仿真获取的55 vol%颗粒增强粘合剂基体复合材料RVE在单轴拉伸(UT)、等双轴拉伸(ET)、纯剪切(PS)下的2方向应力应变曲线,RVE中有50%区域脱粘(即$\bar l = 0.50$)
Figure 3. The 2-directional stress-strain curves obtained by numerical simulation of 55 vol% particle-reinforced binder matrix composites RVE under uniaxial tensile (UT), equibiaxial tensile (ET), and pure shear (PS), with 50% region of debonding in RVE (i.e. $\bar l = 0.50$)
图 4 数值仿真获取的不同程度损伤下55 vol%颗粒增强粘合剂基体复合材料RVE的弹性模量(2方向)(${E_2}$)、体积模量($K$)、剪切模量($G$)散点图
Figure 4. Scatter plots of Young's modulus (2-direction) (${E_2}$), bulk modulus ($K$), and shear modulus ($G$) of 55 vol% particle-reinforced binder matrix composites RVE under different levels of damage obtained by numerical simulation
图 5 含损伤力学本构神经网络训练框架(以变形梯度${\boldsymbol{F}}$和无量纲裂纹长度$\bar l$为输入,名义应力${\boldsymbol{P}}({\boldsymbol{F}},\bar l)$为输出。其中,力学本构神经网络部分以变形梯度${\boldsymbol{F}}$的不变量${\bar I_1}$、${\bar I_2}$、$J$为输入,输出标量自由能函数$\psi ({\boldsymbol{F}})$;损伤折减神经网络部分以无量纲裂纹长度$\bar l$为输入,输出损伤变量$L(\bar l)$。各隐藏层节点设置可训练权重$ {w_{m,n}} $,$m$为单个网络中的隐藏层编号,$n$为隐藏层中的节点编号;$ {f}_{n}(·) $为激活函数,$n$不同则函数不同)
Figure 5. Damage-containing constitutive neural network training framework with deformation gradient ${\boldsymbol{F}}$ and dimensionless crack length $\bar l$ as inputs and nominal stress ${\boldsymbol{P}}({\boldsymbol{F}},\bar l)$ as output (In this framework, the Constitutive neural network part inputs invariant deviation ${\bar I_1}$, ${\bar I_2}$, $J$ of deformation gradient ${\boldsymbol{F}}$,outputs the scalar free energy function $\psi ({\boldsymbol{F}})$. The damage neural network part inputs the dimensionless crack lengths $\bar l$, and outputs the damage variables $L(\bar l)$. Each hidden layer node is set with trainable weights $ {w_{m,n}} $, $m$ is the number of hidden layers in a single network and $n$ is the number of nodes in the hidden layer. $ {f}_{i}(·) $ is the activation function, which is different for different $i$)
图 6 基于变形梯度不变量、自由能的力学本构神经网络训练框架(采用不变量偏差$[{\bar I_1} - 3]$、$[{\bar I_2} - 3]$、$[J - 1]$作为隐藏层的输入变量,第一层隐藏层以线性$ {(·)}^{1} $、二次函数$ {(·)}^{2} $作为激活函数,第二层以恒等函数$ (·) $和指数函数$ (\mathrm{exp}(·)-1) $作为激活函数)
Figure 6. Constitutive neural network training framework based on deformation gradient invariants, free energy theory (The invariant deviations $[{\bar I_1} - 3]$, $[{\bar I_2} - 3]$, $[J - 1]$ are used as the input variables of the hidden layer, the first hidden layer uses linear function $ {(·)}^{1} $ , quadratic function $ {(·)}^{2} $ as the activation function, and the second hidden layer uses the identity $ (·) $ and exponential function $ (\mathrm{exp}(·)-1) $ as the activation function)
图 9 以单一变形数据作训练集,力学本构神经网络模型对未损伤RVE在UT/ET/PS下应力-变形关系的预测结果(其中${R^2}$为预测评估数,${R^2} = 1.00$表示基本吻合)
Figure 9. Prediction of stress-deformation relationship of undamaged RVE under UT/ET/PS by constitutive neural network model using single deformation data as training set (Where ${R^2}$ is the number of predicted evaluations, and ${R^2} = 1.00$ indicates substantial agreement)
-
[1] XING R S, WANG L, ZHANG F T, et al. Mechanical behavior and constitutive model of NEPE solid propellant in finite deformation[J]. Mechanics of Materials, 2022, 172: 104383. doi: 10.1016/j.mechmat.2022.104383 [2] 侯晓, 张旭, 刘向阳, 等. 固体火箭发动机药柱结构完整性研究进展[J]. 宇航学报, 2023, 44(4): 566-579. doi: 10.3873/j.issn.1000-1328.2023.04.011HOU Xiao, ZHANG Xu, LIU Xiangyang, et al. Progress of structural integrity of solid rocket motor pillars[J]. Journal of Astronautics, 2023, 44(4): 566-579(in Chinese). doi: 10.3873/j.issn.1000-1328.2023.04.011 [3] 武晓松, 陈军, 王栋. 固体火箭发动机原理[M]. 北京: 兵器工业出版社, 2010.WU Xiaosong, CHEN Jun, WANG Dong. Principles of Solid Rocket Engine [M]. Beijing: Arms Industry Press, 2010(in Chinese). [4] 强洪夫, 王稼祥, 王哲君, 等. 复合固体推进剂强度、损伤与断裂失效研究进展[J]. 推进技术, 2023, 46(7): 561-588.QIANG Hongfu, WANG Jiaxiang, WANG Zhejun, et al. Advances in strength, damage and fracture failure of composite solid propellants[J]. Propulsion Technology, 2023, 46(7): 561-588(in Chinese). [5] 杜善义, 王彪. 复合材料细观力学[M]. 北京: 科学出版社, 1998.DU Shanyi, WANG Biao. Fine mechanics of composite materials [M]. Beijing: Science Press, 1998(in Chinese). [6] ESHELBY J D. The determination of the elastic field of an ellipsoidal inclusion, and related problems[J]. Proceedings of the Royal Society of London A: Mathematical, Physical and Engineering Sciences, 1957, 241(1226): 376-396. doi: 10.1098/rspa.1957.0133 [7] GURSON A L. Continuum theory of ductile rupture by void nucleation and growth: Part I—Yield criteria and flow rules for porous ductile media[J]. Journal of Engineering Materials and Technology, 1977, 99(1): 2-15. doi: 10.1115/1.3443401 [8] DUAN H L, WANG J, HUANG Z P, et al. Size-dependent effective elastic constants of solids containing nano-inhomogeneities with interface stress[J]. Journal of the Mechanics and Physics of Solids, 2005, 53(7): 1574-1596. doi: 10.1016/j.jmps.2005.02.009 [9] SHEN W Q, ZHANG J, SHAO J F, et al. Approximate macroscopic yield criteria for Drucker-Prager type solids with spheroidal voids[J]. International Journal of Plasticity, 2017, 99: 221-247. doi: 10.1016/j.ijplas.2017.09.008 [10] M WUBULIAISAN, WU Y Q, XIAO H, et al. Multiscale viscoelastic constitutive modeling of solid propellants subjected to large deformation[J]. International Journal of Solids and Structures, 2023, 262: 112084. [11] CANGA M E, BECKER E B, ÖZÜPEK Ş. Constitutive modeling of viscoelastic materials with damage – computational aspects[J]. Computer Methods in Applied Mechanics and Engineering, 2001, 190(15-17): 2207-2226. doi: 10.1016/S0045-7825(00)00231-0 [12] XU F, ARAVAS N, SOFRONIS P. Constitutive modeling of solid propellant materials with evolving microstructural damage[J]. Journal of the Mechanics and Physics of Solids, 2008, 56(5): 2050-2073. doi: 10.1016/j.jmps.2007.10.013 [13] LEI M, WANG J J, CHENG J M, et al. A constitutive model of the solid propellants considering the interface strength and dewetting[J]. Composites Science and Technology, 2020, 185(1): 107893. [14] LI Z, LEI M, KOU Q Q, et al. A multiscale viscoelastic constitutive model of unidirectional carbon fiber reinforced PEEK over a wide temperature range[J]. Composite Structures, 2023, 321: 117258. doi: 10.1016/j.compstruct.2023.117258 [15] KARNIADAKIS G E, KEVREKIDIS I G, LU L, et al. Physics-informed machine learning[J]. Nature Reviews Physics, 2021, 3(6): 422-440. doi: 10.1038/s42254-021-00314-5 [16] HERNÁNDEZ Q, Badías A, Chinesta F, et al. Thermodynamics-informed neural networks for physically realistic mixed reality[J]. Computer Methods in Applied Mechanics and Engineering, 2023, 407: 115912. doi: 10.1016/j.cma.2023.115912 [17] REZAEI S, HARANDI A, MOEINEDDIN A, et al. A mixed formulation for physics-informed neural networks as a potential solver for engineering problems in heterogeneous domains: Comparison with finite element method[J]. Computer Methods in Applied Mechanics and Engineering, 2022, 401, Part B, 115616. [18] BRODNIK N R, MUIR C, TULSHIBAGWALE N, et al. Perspective: Machine learning in experimental solid mechanics[J]. Journal of the Mechanics and Physics of Solids, 2023, 173: 105231. doi: 10.1016/j.jmps.2023.105231 [19] BAI J H, LIU G R, GUPTA A, et al. Physics-informed radial basis network (PIRBN): A local approximating neural network for solving nonlinear partial differential equations[J]. Computer Methods in Applied Mechanics and Engineering, 2023, 451: 116290. [20] LINKA K and KUHL E. A new family of Constitutive Artificial Neural Networks towards automated model discovery[J]. Computer Methods in Applied Mechanics and Engineering, 2023, 403: 115731. doi: 10.1016/j.cma.2022.115731 [21] MARINO E, FLASCHEL M, KUMAR S, et al. Automated identification of linear viscoelastic constitutive laws with EUCLID[J]. Mechanics of Materials, 2023, 181: 181104643. [22] WUBULIAISAN M, WU Y, and HOU X. A unified viscoelastic model of progressive damage and failure for solid propellants[J]. International Journal of Plasticity, 2023, 170: 103765. doi: 10.1016/j.ijplas.2023.103765 [23] LEI M, CHEN E H, ZHAO Z, et al. A Temperature/Strain-Rate Dependent Finite Deformation Constitutive and Failure Model of Solid Propellants[J]. SCIENCE CHINA Physics, Mechanics & Astronomy, 2023, 66(9): 294611. [24] 赵亚溥. 近代连续介质力学[M] . 北京: 科学出版社, 2016.ZHAO Yapu. Modern mechanics of continuous media [M]. Beijing: Science Press, 2016(in Chinese). [25] COLEMAN B D and GURTIN M E. Thermodynamics with Internal State Variables[J]. The Journal of Chemical Physics, 1967, 47(2): 597-613. doi: 10.1063/1.1711937 [26] GERHARD A HOLZAPFEL. Nonlinear Solid Mechanics[M]. Wiley publisher. 2000. [27] L M KACHANOV. On time to rupture in creep conditions (in russian) Izviestia Akademii Nauk SSSR[J]. Otdelenie Tekhnicheskikh Nauk, 1958, 8: 26-31. [28] MURAKAMI S. Continnum Damage Mechanics [M]. Springer. 2012. [29] LEMAITRE J. A continuous damage mechanics model for ductile fracture[J]. J. Eng. Mater. Technol, 1985, 107: 83-89. doi: 10.1115/1.3225775 [30] MURAKAMI S. Mechanical Modeling of Material Damage[J]. ASME Journal of Applied Mechanics, 1988, 55: 280-286. doi: 10.1115/1.3173673 [31] HUR J, PARK J B, JUNG G D, et. al. , Enhancements on a micromechanical constitutive model of solid propellant[J]. International Journal of Solids and Structures, 2016, 87: 110-119. [32] RIVLIN R S and SAUNDERS D W. Large elastic deformations of isotropic materials VII. Experiments on the deformation of rubber[J]. Philosophical Transactions of the Royal Society of London. Series A, Mathematical and Physical Sciences, 1951, 243: 251-288. [33] LEI M, HAMEL C M, YUAN C, et al. 3D Printed Two-Dimensional Periodic Structures with Tailored In-Plane Dynamic Responses and Fracture Behaviors[J]. Compos. Sci. Technol, 2018, 159: 189-198. doi: 10.1016/j.compscitech.2018.02.024 [34] CAI C, WANG B, YIN W L, et al. A new algorithm to generate non-uniformly dispersed representative volume elements of composite materials with high volume fractions[J]. Materials & Design, 2022, 219: 110750. [35] BALL J M. Convexity conditions and existence theorems in nonlinear elasticity[J]. Arch. Ration. Mech. Anal, 1977, 63: 337-403. [36] HARTMANN S, NEFF P. Polyconvexity of generalized polynomial-type hyperelastic strain energy functions for near-incompressibility[J]. Solids Struct, 2003, 40: 2767-2791. doi: 10.1016/S0020-7683(03)00086-6 [37] PAN H, YANG J, SHI Y, et al. BP neural network application model of predicting the apple hardness[J]. Journal of Computational and Theoretical Nanoscience, 2015, 12(9): 2802-2807. doi: 10.1166/jctn.2015.4180 [38] SAID BASSAM EL. Predicting the non-linear response of composite materials using deep recurrent convolutional neural networks[J]. International Journal of Solids and Structures, 2023, 276: 112334. doi: 10.1016/j.ijsolstr.2023.112334 [39] Mooney M. A theory of large elastic deformations[J]. Journal of Applied Physics, 1940, 11(9): 582-592. doi: 10.1063/1.1712836 -
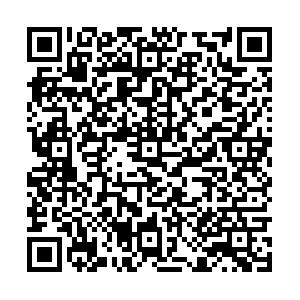
计量
- 文章访问数: 127
- HTML全文浏览量: 59
- 被引次数: 0